
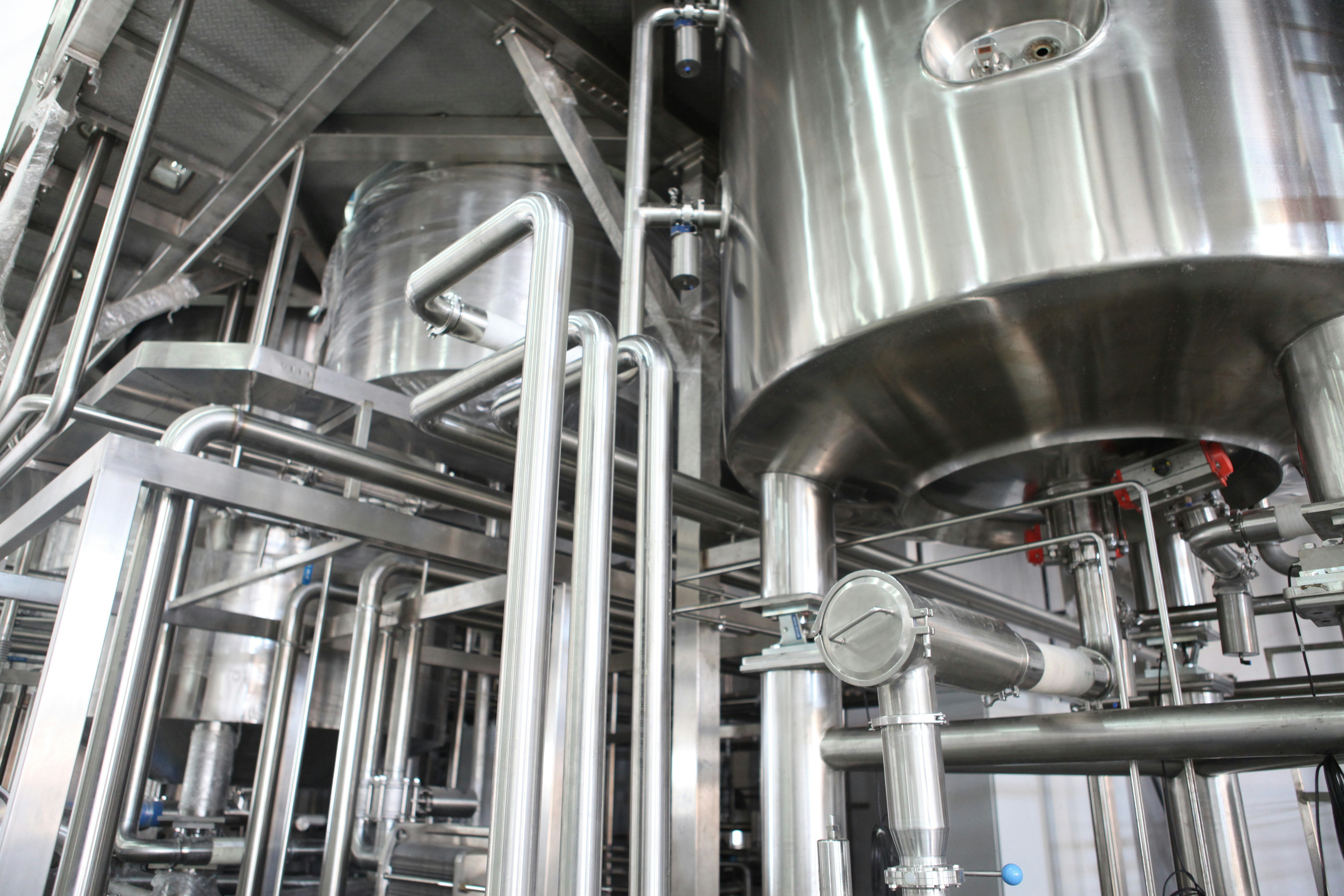
From Dynamics to Relationships in Data.
As modern systems grow in complexity, monitoring their health becomes increasingly critical. Traditional methods typically analyze the dynamics of each individual time series or their immediate context. However, this neglects a crucial aspect: the complex relationships that exist between these time series.
A system's dynamics are influenced not only by individual components but also by how it's operated, controlled, and impacted by external factors. This makes it difficult for methods focused on dynamics modelling to generalize to new operating conditions. The key lies in understanding the interconnected nature of the data. When a fault occurs, it disrupts the usual relationships between time series. Monitoring these changes offers a more reliable approach to fault detection and diagnosis.
But the potential of relational data extends far beyond fault detection. By leveraging the rich information hidden within these relationships, we unlock a wealth of possibilities, including virtual sensing, sensor state estimation, and imputation.